Business is increasingly data-driven. Many companies have famously bragged about creating a data-driven culture, but can data analytics truly impact business competitiveness? Is it real? Let’s see what some industry analysts have to say on the topic.
Some say that without examining the potential business impacts of data analytics and adjusting business strategy accordingly, organizations risk losing a competitive advantage.[1]
IDC predicts that, “By 2020, 80% of enterprises will create data management and monetization capabilities, thus enhancing enterprise functions, strengthening competitiveness, and creating new sources of revenue.”[2]
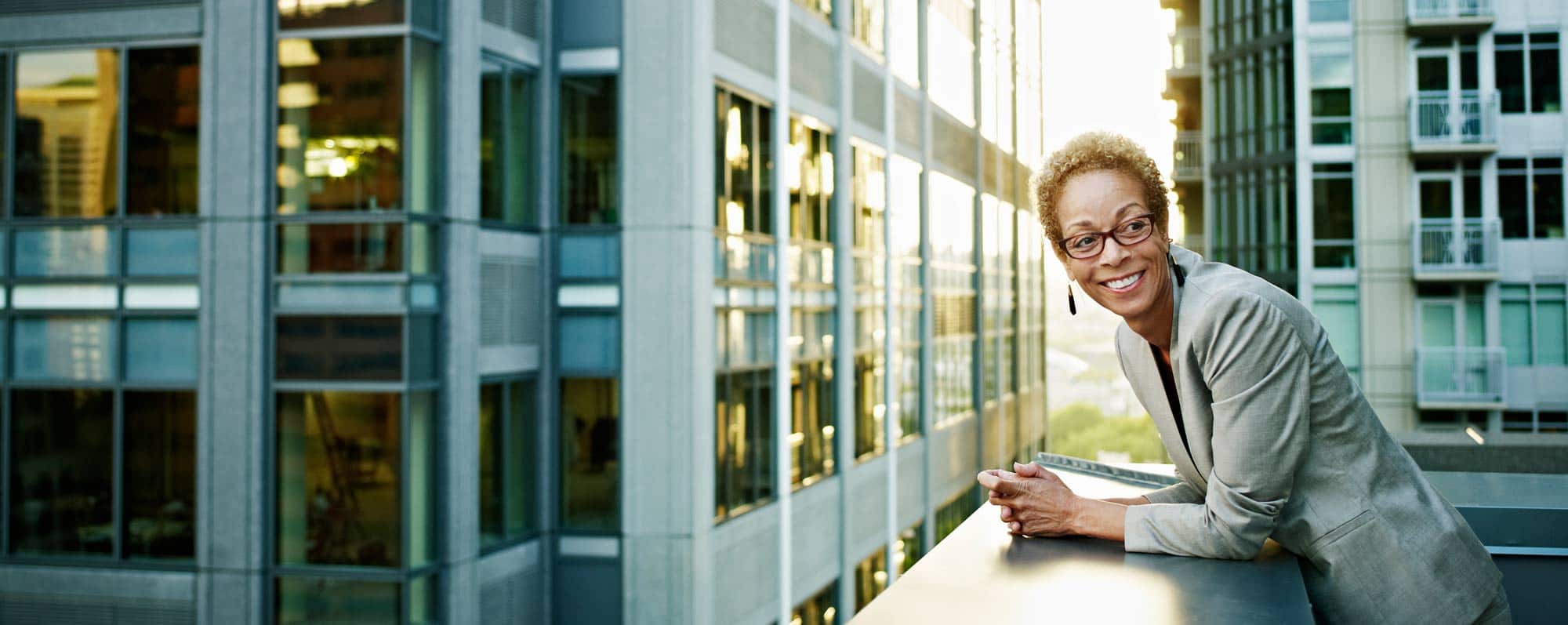
Data analytics isn’t a dark art, but it does take some work. Consider these different ways of leveraging data analytics to drive your business forward:
Way #1 – Improve Execution of Existing Processes
The industry analysts quoted above touch on two different aspects of analytics impact on business competitiveness: 1) executing better and 2) finding new sources of revenue.
Improving execution of existing processes tends to be a lower risk effort that can also bear speedy results. This might include getting a better view of your supply chain, or improving manufacturing operations. It can also include getting a better understanding of your customers and their behavior. Improving your existing business operations is a great way to get started since you’re leveraging what you know already works, and allows you to make it work better.
Improving your existing business operations is a great way to get started.
Way #2 – Create New Business Models and Revenue Streams
It has often been said that the best valuations for startups goes to companies that can create new business models. If you have the imagination to re-envision your business with a new business model, or better yet, a new way to monetize data – you may have found a significant business differentiator. This is much harder to do task than improving the existing model, but the payoff can be much more long-lasting.
Way #3 – Get Accurate Results With More Data
Analytics depends on data and algorithms, and although it may not be obvious, most data scientists believe that more data is more important than better algorithms. The simple reason is that you need a lot of data to train algorithms. The more data you can leverage, and the more diverse it is, will yield better results for machine learning.
By looking at a wide variety of relevant data, algorithms may find relationships that a smaller data set may not. Also, it is hard to identify anomalies or understand underlying relationships, bias and variance if you don’t have enough data to compare. The difference can be a competitive advantage, or worse, failure.
Way #4 – Find Additional Sources of Valuable Data
Finding data that can be useful takes some work. Indeed, most of the work that is required to support an analytics effort is in the identification, aggregation, augmentation, cleansing and labeling of data before you start to use it in your analytics job (here’s a great view into 3 simple yet critical challenges of data analytics in machine learning).
Don’t forget that external data can be useful.
When looking for relevant data consider all internal sources of data- don’t skip departmental siloes and historic data that may still be on tape as well as the data already in your data center. Don’t forget that external data can be useful also- consider census data, weather data or other public or purchasable data to support your needs. The concept of IoT data marketplaces is not far from becoming mainstream.
Way #5 – Architect a Better Data Infrastructure
Data analytics requires an understanding of how data is served, accessed and processed at every step of the pipeline. Make sure you have the infrastructure to support this effort (not sure what that means – read this).
Training an algorithm is an iterative process, so more iterations generally lead to better algorithms. Combine that with a large amount of data, longer retention periods (potentially forever) and a need for faster results and you’ll see that you need to have the right infrastructure to make it all work.
Improve Your Data Analytics Strategy – It’s Not Too Late
Increasing your data analytics efforts is not just important, it’s a matter of survival. The good news is, it’s not too late – Gartner shows that 87% of organizations have low business intelligence and analytics maturity.[3] So, you’re not alone in figuring it out, but it’s certainly time to tackle it before your competition speeds past you with their data-driven insights.
I’d like to invite you to join me on May 29 at 10:00am Pacific Daylight Time when I’ll dive into how to develop a data strategy for analytics. I hope to see you there!
Save your seat/stream: How to Develop a Data Strategy for Analytics
[2] https://www.businesswire.com/news/home/20181107005026/en/Global-Leader-Digital-Transformation-Market-Research-IDC
[3] https://www.gartner.com/en/newsroom/press-releases/2018-12-06-gartner-data-shows-87-percent-of-organizations-have-low-bi-and-analytics-maturity