Digital twins are helping manufacturing-centric companies replicate physical environments virtually. We should know, as a company that produces millions of devices across our HDDs and flash-based media each year. This virtual tool is a way to study manufacturing processes, identify bottlenecks, and run various tests with reduced real-world overhead. The end goal is to make our operations more efficient by improving throughput and cutting down on expenses.
In this blog post, I’ll give you an introduction to digital twins and highlight how we are using them to improve upon our system-on-a-chip (SoC) manufacturing.
Our Definition of a Digital Twin
Simply put, a digital twin is a virtual copy of a physical entity. A digital twin uses software to simulate down to the individual devices, tools, processes, systems, and even people or locations. Doing so avoids the expenses, resources, potential risks and time for real-world experiments – though real-world testing is needed to validate the results of digital twins.
Some of the world’s largest manufacturers, from airplane manufacturers to data storage providers, have embraced digital twins as a way to increase productivity in their assembly lines, improve product design, and accelerate product testing through virtual environments. There’s even hope of rebuilding the 850-year-old Notre Dame Cathedral point by point through a digital twin.
What Challenges Can Digital Twins Help Solve?
Digital twins are being used in a variety of applications. One important use case has been in improving manufacturing operations. By using digital twins, companies can mimic their smart factories, identify bottlenecks (across components, systems, processes, and other assets), test potential solutions, and estimate the results of interactions between components and stochastic changes that may happen during operations – all in a virtual environment. This simulation saves organizations from spending time, resources, and expenses to test their operational hypotheses “in the wild”.
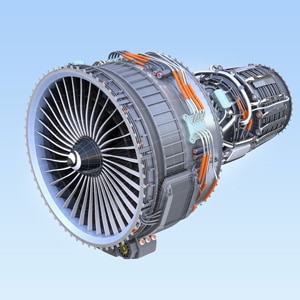
Another use for digital twins has been in product design and testing. Take a jet engine, for example. Thousands of individual components are needed to build an engine for one of the world’s most popular airliners1, along with extensive safety testing. Rather than constructing an expensive, real-world engine, a digital twin can be built to create a 3D replica of the airplane engine. Then, the virtual engine can be analyzed, examined, and tested according to proper specifications. A digital twin could also be created for an existing airplane engine to suggest predictive maintenance through component analysis.
“Copy and Pasting” an Entire Fab Floor in Shanghai
Digital twins help us simulate the factory operations, predict, and account for expected cycle time variations to meet dynamic changes in flash storage demand. Using the blueprint of our semiconductor fabrication plant (or “fab”) in Shanghai, China, we created a digital twin of the second floor of the facility. This area includes our Autonomous Robot Vehicles (ARVs) and end-of-line test equipment machines, or “testers”, for flash-based, system-on-a-chip (SoCs).
Our approach was to use a combination of operational research and stochastic Q-time theory to create a historically accurate digital twin. This data was split into two parts: historical waiting and service time distribution of Device under Test (DUT) at the testers, and ARV movement distributions in our factory. We calculated the expected amount of service time for a given robot to transfer a test unit – a SoC, in this case – to a given test machine. Our goal was to optimize the overall service time, and thus increase our throughput and efficiency on the factory floor.
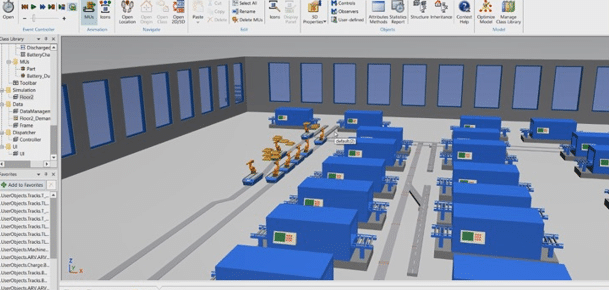
12-Week Cycles of Digital Twin Experimentation
Many of my team’s digital initiatives run in 12-week intervals, which we call a “Rapid Learning Cycle”. We spend twelve weeks experimenting, tinkering with the various inputs to the digital twin, and analyzing the expected outputs. Ten weeks into this period, we evaluate the experimental results and decide whether to go-ahead with a full roll out, or to cut the project.
Digital initiatives that make the cut continue as “pilots” for another 6 – 12 weeks. In the case of our ARV digital twin, the pilot was implemented in stages over six weeks with an incremental release of business decision rules learned from the digital twin. We use a gradual approach to avoid unexpected problems or issues during rollout. The new, upgraded ARV routing rules have been fully operational since this past January.
Digital Twins Lead to 99 Percent ARV Automation
By using digital twins to optimize our factory floor operations, the ARV utilization rate for pick and place of jobs by our service robots has gone up by 25 percent. We created multiple service zones in the shop-floor and positioned ARVs in specific zones; this helped reduce overall travel time. We’ve also created specialized functions for each robot: some are used exclusively for loading test machines, while others are used to unload testers.
The digital twin has now enabled 99 percent automation in the use of our ARVs. Finally, we used digital twins to determine the impact of adding additional robots to our factory floor. By estimating how a robot will impact the service line, we can scale up quickly and effectively.
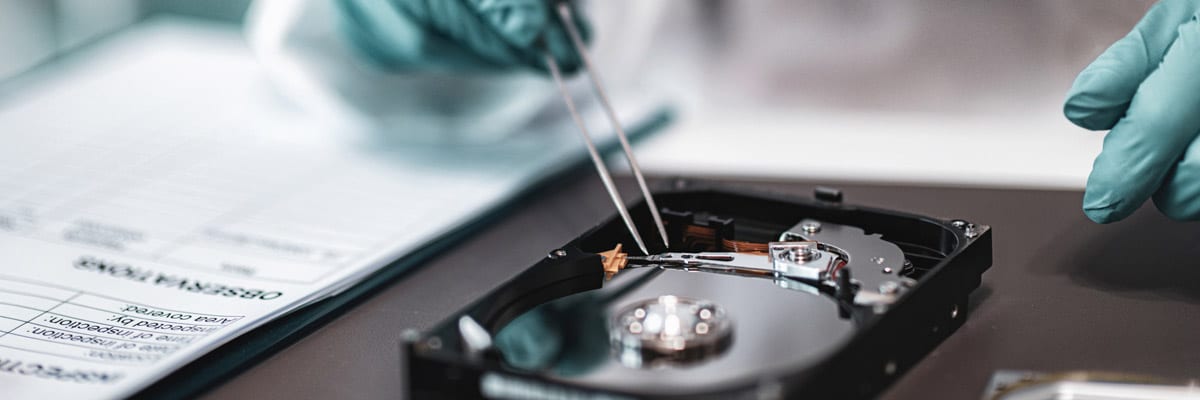
Bringing Digital Twins to Our HDD Ops
Looking ahead, we’re using this same digital twin framework to optimize throughput for our HDD operations. Specifically, we want to use quantitative and qualitative analysis in our HDD assembly line. Our goal is to reduce cycle time and optimize capital expenditure spending through the right mix of line capacity parameters and performance. Given our current results with digital twins, I’m hoping that we “see double” in our future initiatives!
Learn More
- Find out how we’re using data toward a paperless supply chain >
- Explore our IIoT products, purpose-built for Industry 4.0 >
- See our IoT and edge solutions >
Sources:
- Jet Engines | Encyclopedia.com. https://www.encyclopedia.com/science-and-technology/technology/aviation-general/jet-engine