Not everyone agrees on what the future of healthcare will look like. For some, the future involves hacking genes like software, for others Artificial Intelligence (AI) in healthcare is more cautionary, yet no less optimistic.
Working with various research institutes, I want to add a third perspective to the discussion looking at the technologies that will deliver better living, early prediction, and precision medicine, and the key trends on our path to breakthrough.
1. Beyond the Constraints of Human Knowledge
Recently, Artificial Intelligence became the Go game world champion, and there’s a critical lesson learned. The previous champion, also software, beat the human world champion by leveraging more than 100,000 Go games as its knowledge base. In contrast, the new and more powerful version of the software was programmed only with the basic rules of Go. Everything else it learned from scratch by playing against itself.
Why is this difference so monumental? The first program was constricted to known human strategy. Once we allowed the machine to learn for itself, it removed the constraints of human knowledge and could in fact be more powerful.
2. We Often Don’t Even Know What We’re Looking For
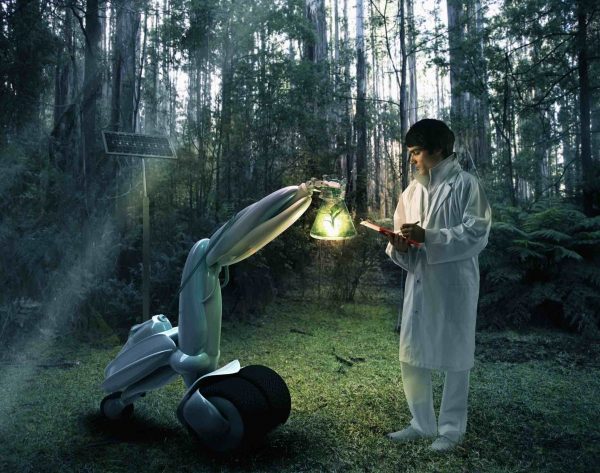
“The DNA sequence of an individual can be accessed, but we still can’t explain why one gene is related to a particular disease.” In the interview, Geneticist Nir Barzilai speaks of the great truth of much of the current research, particularly in genomics: researchers don’t always know what they are looking for. We still don’t really understand much about genes.
The hope is that Machine Learning will be able to not only find answers, but also find the questions we are looking to ask. The approach may be similar in some sense to the Go game example. Humans can provide rules for healthy genes, proteins etc. and examples of mutated genes. Machine Learning will find better approaches in looking for patterns for commonalities or non-commonalities and eventually learn to recognize incomplete, patchy, and glitch-riddled genomes that introduce errors at every step of the way.
3. You Can Massively Produce Machines, Not Doctors.
Like in the game Go, the intelligence part of AI in healthcare is very narrow. But if you train Machine Learning to do/recognize a specific task, it can be very powerful, particularly in places where there is not enough medical expertise.
A good example is a recent development in the detection of diabetes-related eye disease, diabetic retinopathy, which can cause blindness if untreated. The patient does not sense early symptoms, so an expert with a specialized device is needed to examine images of the retina for early signs.
Now, Machine Learning has been trained, through image recognition, to spot early stages of the disease. If low-cost machines can be distributed to areas where there is a lack of ophthalmologists and medical experts, it can help millions of diabetes patients get an annual screening for diabetic retinopathy, and possibly prevent many from becoming blind. You can massively produce machines, but not eye doctors.
4. Data – The Source of the Problems (and Solutions)
“We have so much biological data that we’re pulling in from so many places. Now we have to figure out how to weave all the data together, so we can learn how one bit of data works with the other.” Geneticist Nir Barzilai hit the nail on the head on probably the most critical work we are doing with many of our customers.
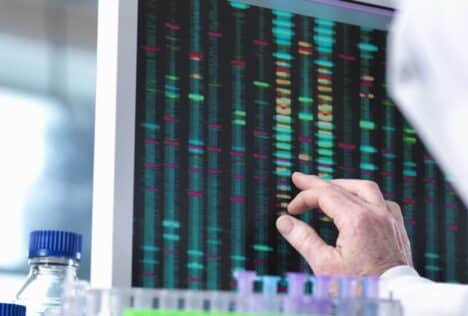
Imaging techniques are improving drastically; file sizes are moving from gigabytes into the terabytes and data sets from terabytes to petabytes. In life sciences, the more data you have, and the more diverse that data, the higher the accuracy of your results. Machine Learning needs right and wrong data to train the machine. Without enough data your model is worthless. As such, Machine Learning workflows need to be incredibly scalable, reliable and economical. The data lifecycle requires huge repositories of images and data to draw from as well as fast processing for analysis and model building using samples of that data.
The introduction of object storage and the cloud have changed this workflow dramatically. Institutions can take advantage of object storage on premises for the bulk of their data (petabytes and petabytes of data) in a much more cost efficient infrastructure than previous NAS systems or the public cloud. Furthermore, object storage enables scientists to curate their data by storing metadata as additional tags along with the data, and keeping data on premises permits working with sensitive patient information in a private system. The introduction of NVMe™, a new standardized storage protocol for accessing high-speed storage media, together with GPUs, is the fastest way to load data for learning models.
Yet this is just one part of the equation. For scientific research, data can’t remain siloed. Organizations need to combine research data pools. They must leverage cloud services and resources, whether in the community cloud or the public cloud, to collaborate at scale. That brings up the next key trend and perhaps the biggest challenge yet – data security.
5. How do You Protect Human Data?
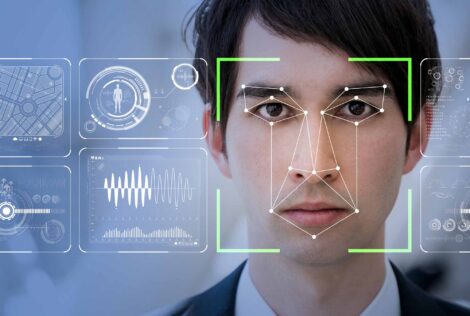
The bad news about genes is data security. If someone steals a credit card or social security number you can get it replaced. But what about your DNA? Once we have the intelligence to map out potential disease, how can we guard that data? What will your rights be if your insurance company decided you are a high risk customer, or your DNA shows you have no potential for a particular job?
The questions of privacy, security and encryption are critical ones even before we reach further advancements for AI in healthcare. Already today people are using direct-to-consumer genetic testing to find out their ancestry through their DNA. But who owns that data, how is it guarded and what happens if it’s hacked or sold?
For AI in healthcare to truly transform medicine and patient experience, these are the key questions the industry will have to answer.
Research Data Should Live Forever
Western Digital moves mountains of data through the bioinformatics workflow. We are pioneering advancements in HDDs, SSDs, platforms and fully featured object and unified flash storage systems, with innovation up and down the stack that is unmatched.
Want to learn how we can accelerate your innovation? Meet us at the Big Science Business Forum 2018, 26-28 February 2018 in Copenhagen, Denmark and at the BioInformatics Strategy Meeting on the 5th of March in Zurich, Austria.
Join us live online on March 22 at 10:00 am PT to learn about Globus for ActiveScale™, a cost-effective solution for on-premise object storage that’s simple to deploy and use.