Derek Stewart: Material Frontiers in the Age of AI
Derek Stewart is the resident theorist for emerging non-volatile memory in Western Digital’s R&D. He is an expert in the computational modeling of new materials and nanostructures, taking the theoretical to the tangible.
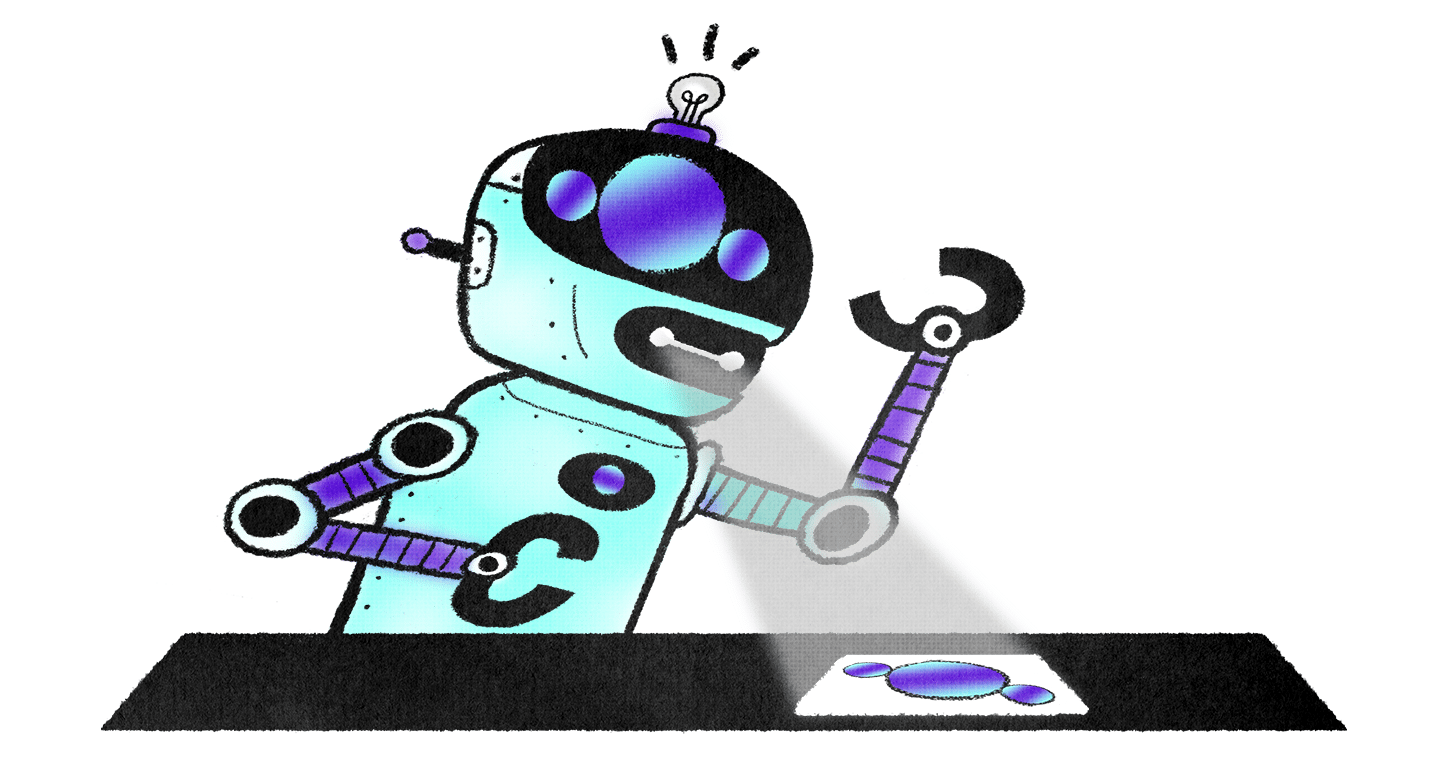
How much do we, as in human civilization, know about materials?
We know quite a bit about materials. We’re at the point where we can construct devices that are a combination of deposited nanoscale material layers, some just angstroms thick, and it’s fairly routine. But I would say that the challenge is that we’re still learning about how materials interact with each other.
Is this where AI and computation come in?
Yes, to some extent. We are now reaching what I see as a golden age for computational materials science. There’s a convergence of cheap, fast computers with large memory capacity, robust theoretical approaches like density functional theory, and the introduction of efficient parallel computing algorithms that can tackle quantum-mechanical calculations—a perfect confluence for large-scale computational material searches.
Where do you see machine learning being the most impactful?
For a long time, developing atomic potentials, which describe how atoms experience force between them, was a sort of dark art. There were people who developed analytical expressions to describe these potentials, and they would work on optimizing them for particular situations. But every time you add in a new element, you need somebody to come in and figure out how that element is going to interact with the other elements in the system. That has been the big roadblock.
The big advance with machine learning is that we can now do a limited set of first principles calculations that are very good at predicting interactions between atoms. And in a similar way that we can train a computer to recognize an image of a cat, we can train it with this dataset and then transfer that accuracy to much larger-scale calculations.
If 70 years ago we could simulate a single atom or two, we’re now getting to the scale where we can simulate close to a million atoms using machine learning-based molecular dynamics. It really opens up the size of the atomistic system we can look at and what that means for understanding material interfaces.
Okay, let’s flip the question. What are the limitations of AI in material discovery?
Since this is a young field, I think we’re working to get to the point on how to judge AI’s work and how to improve its results. If you’re reviewing a paper based on machine learning techniques, there are a lot of things that are happening in that black box. How do you evaluate these promising results if you can’t see under the hood?
I would say, overall, it can help in identifying trends, but we need to be careful that we’re not assuming that these models can give us exact answers.
Recently, there were big announcements about AI discovering millions of new materials—something that would have taken humans hundreds of years to arrive at alone. How excited should we be?
I think we should be cautiously optimistic. We need to view these searches as another tool to identify promising areas for future exploration, but it’s also important to note that a lot of these materials do not exist in nature, and it can take time to either verify or disprove these theoretical predictions. It’s not going to solve all of society’s problems tomorrow, but it is informing new material areas to explore.
How do you parse the materials with real potential from science-fiction fodder? What are you looking for or testing?
I would say one of the greatest challenges is gauging how stable that new material is going to be at room temperature and how they’re going to interact with their environment. If it doesn’t pass those checks, it doesn’t mean that the material isn’t interesting or worth exploring further, but it can highlight some challenges in going from that material concept to something that you can hold in your hand or use in a device.
“We need to make sure that any new material we work with checks all those boxes, which requires a sustained investment of time and capital. Even then, that’s not a guarantee of success.”
What does that mean for your work? What’s the path from discovery to product?
There are a lot of technological barriers that you must overcome between the discovery of a material effect and implementing it in a product at scale.
For any new memory device we consider, it’s got to have nanoscale dimensions for high memory density, and fast switching speed so it can compete in the marketplace. We have high endurance so that it can last a long time for the customers and good retention so they don’t lose their data. And then you have other things to consider like low power and wide operating temperature range.
We need to make sure that any new material we work with checks all those boxes, which requires a sustained investment of time and capital. Even then, that’s not a guarantee of success.
There’s a term for technologies that get about halfway and then never really come to fruition, referred to as the Valley of Death, where progress on a particular concept can falter. If the dominant technology is good enough, there isn’t as much of an incentive to invest in these new technologies and the products never materialize.
This Valley of Death speaks to the energy, time, and investment it takes to make a product a reality. Despite that labor-intensive effort, we’re still searching for new materials. Why? Why are we still looking for new materials instead of developing the ones we already discovered? What are we hoping to discover?
We are getting to a point where we can see Moore’s Law not stopping, but maybe slowing down, because we are reaching the physical limits of how far we can shrink these devices. At the same time, we’re starting to see more specialization and differentiation of hardware.
Some researchers have likened this to the Cambrian explosion and the development of life on the Earth. With technology today, you could argue going with the single-cell Moore’s Law approach is no longer good enough for every situation. And so we need to kind of expand and diversify and evolve to address specific initiatives and problems, and new materials may be more important in tackling this.
It’s interesting you mention Moore’s Law, because it’s so interconnected with materials. How would you describe this relationship?
Materials research is essential for Moore’s Law, and there are several historical examples where materials breakthroughs were required to keep up with Moore’s Law. For example, with integrated circuits, there was a transition from aluminum to copper interconnects, reducing electron migration and increasing the conductivity of the interconnect lines.
We also see this in Western Digital’s flash industry; constantly pushing down the cell dimension size for the two-dimensional flash and the ability to create 3D NAND structures relies on a lot of advances in terms of material deposition and processing.
But there’s also this interesting meeting point we’ve arrived at. On one hand, Moore’s Law is pushing the size of things down further and further. At the same time, Moore’s Law has enabled us to do fast calculations at larger and larger scales. So we’re getting to the point where the simulations can exist on the same scale as the devices, which is exciting.
How important has the semiconductor industry been in pushing our understanding of materials?
The semiconductor and the memory industry have really been key drivers of materials science at the nanoscale. All of the work to maintain Moore’s Law—research into materials deposition, photolithography, characterization, and integration—was just a huge investment in understanding these materials better. Silicon is probably the most researched material in human history.
I think that work and some of the tools that have been developed for this field spill over into other areas. Biological research, battery development, and even quantum computing are going to lead to some exciting new material innovations and maybe even some new material revelations working at those low temperatures. I also think about glass, which might be one of the oldest forms of material research, but one that continues to surprise and pay huge dividends to society.
As new materials emerge, do you see the industry moving beyond silicon?
If I look at modern society, we are probably in the age of silicon and lithium, because those are the two key components of the small computers or cell phones that we all carry around with us every day. And without those two elements, society as we know it would not exist.
I don’t think silicon is ever going to get replaced because we have developed such a large database of knowledge about silicon and how other materials interact with it. There is still a small army of engineers working on improving silicon. It would really have to hit an extreme roadblock for something to supplant it.
So what does the future look like?
It’s a tough question to predict what the future of materials will be. But as it becomes easier to fabricate low-power connected devices, I think you will see greater integration of sensors into ordinary household and office items. Some of these devices may even be able to harvest energy from vibrations or Wi-Fi signals that are permeating the rooms. Many of these devices and sensors will also rely on neuromorphic analog signal processing to help improve efficiency and reduce power needs.
I also think you will see things like flexible electronics progressing and integrated into clothing to provide health diagnostics and fashion options. You can look at some other areas that are promising for the future such as 2D materials—single atomic sheets, like graphene, that could be used for future atomic layer-scale electronics.
But how you’re going to take some of these new materials and then integrate them into kind of more industry-scale devices is the biggest challenge. So, I envision the advances in material technology being pervasive, but maybe not so in your face. The future of material science is something more subtle.